When embarking on the journey of a dissertation or capstone project, one of the most crucial and challenging aspects lies in the realm of data analysis. As the saying goes, "knowledge is power," and understanding how to effectively decode and interpret the data you have gathered is essential to unlocking the true potential of your research. Dissertation data analysis is a process that not only provides valuable insights into your study but also serves as the foundation for drawing meaningful conclusions and shaping the future direction of your academic inquiry.
In today’s data-driven world, researchers are equipped with an abundance of information at their fingertips. However, it is the ability to extract pertinent insights, uncover patterns, and identify trends that sets apart successful dissertation projects from the rest. Dissertation data analysis allows scholars to make sense of the vast amount of raw data they have collected, transforming it into a cohesive narrative that tells a compelling story. By employing various statistical techniques, data visualization tools, and qualitative analysis methods, researchers can identify patterns, evaluate hypotheses, and gain a deeper understanding of their research questions.
Importance of Dissertation Data Analysis
Dissertation data analysis is an essential aspect of research that holds tremendous significance in the academic world. It allows researchers to draw meaningful conclusions from extensive datasets, providing valuable insights into their chosen field of study. By employing robust data analysis techniques, researchers can unravel complex patterns, trends, and relationships within their collected data. This enables them to address research questions, support or refute hypotheses, and contribute to the existing knowledge base.
One of the primary reasons why dissertation data analysis is crucial is that it adds credibility to the research findings. By analyzing the data meticulously, researchers can validate their claims and ensure that their results are sound and reliable. This is especially important in the context of dissertations and capstones, where original research is conducted to make a unique contribution to the field. Thorough data analysis helps to establish the credibility of the research, enhancing its validity and trustworthiness.
Moreover, dissertation data analysis plays a critical role in the advancement of knowledge. By examining and interpreting data, researchers can identify patterns and trends that were previously unknown or undiscovered. This process of uncovering hidden insights contributes to the existing body of knowledge, expanding the understanding of a particular subject area. Additionally, data analysis also allows researchers to explore new avenues of research, generating further interest and inquiry.
Furthermore, the insights gained from dissertation data analysis can have practical implications. The findings can be used to inform decision-making processes, shape policies, and improve practices within various domains. For example, in the field of healthcare, data analysis can reveal effective interventions or highlight areas for improvement in patient care. Similarly, in the business sector, data analysis can guide strategic decision-making by identifying consumer trends or optimizing operational processes. In this way, dissertation data analysis has the potential to bring about tangible benefits and positive change in both academia and the real world.
In conclusion, the importance of dissertation data analysis cannot be overstated. It not only adds credibility to the research but also contributes to the advancement of knowledge and has practical applications. By delving into the data and uncovering meaningful insights, researchers can make significant contributions to their field of study and bring about positive change.
Common Data Analysis Methods
When it comes to conducting data analysis for dissertations and capstone projects, researchers employ various methods to derive meaningful insights from their data. In this section, we will explore some commonly used data analysis methods.
- Descriptive Analysis:
Descriptive analysis aims to summarize and describe the main characteristics of the data under study. Researchers often utilize statistical measures such as mean, median, mode, and standard deviation to provide a clear understanding of their data. Through descriptive analysis, researchers can gain insights into the central tendency, variability, and distribution of their dissertation data.
- Inferential Analysis:
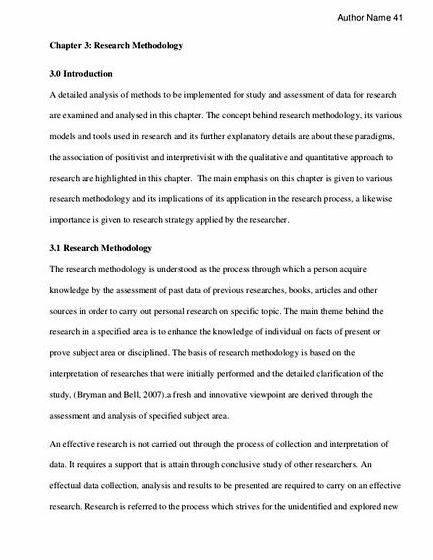
Inferential analysis is a method that allows researchers to draw conclusions and make inferences about a larger population based on a sample of data. Through techniques such as hypothesis testing and estimation, researchers can determine the significance of relationships and differences observed in their dissertation data. This helps generalize the findings to a broader context.
- Help With Dissertation Proposal
- Content Analysis:
Content analysis involves systematically categorizing and analyzing qualitative data such as text, audio, or visual content. Researchers apply predefined coding schemes or develop new codes to identify patterns, themes, and trends within their dissertation data. Content analysis provides a structured approach to interpret and extract meaningful information from unstructured data sources.
By leveraging these common data analysis methods, researchers can unlock the potential of their dissertation data and gain valuable insights. It is important to choose the most appropriate method(s) that align with the research objectives and ensure the validity and reliability of the findings.
Challenges and Tips for Effective Data Analysis
One of the biggest challenges in dissertation data analysis is ensuring the accuracy and reliability of the data. Oftentimes, researchers may face issues such as missing data, outliers, or inconsistent data formats. To overcome these challenges, it is essential to carefully clean and preprocess the data before starting the analysis. By checking for errors and inconsistencies, researchers can ensure the integrity of their findings and draw more valid conclusions.
Another challenge in data analysis is the selection of appropriate statistical methods. With a plethora of techniques available, it can be overwhelming to choose the most suitable one for a particular research question. It is crucial to have a good understanding of different statistical methods and their assumptions to make informed choices. Consulting with statisticians or mentors can also be helpful in selecting the right analytical approach.
Furthermore, a lack of computational skills can hinder effective data analysis. Many researchers may struggle with handling large datasets or performing complex calculations. Learning relevant programming languages, such as R or Python, can significantly enhance one’s ability to manipulate and analyze data efficiently. Additionally, utilizing specialized software and tools designed for data analysis can streamline the process and make it more manageable.
In summary, the challenges in dissertation data analysis include ensuring data accuracy, selecting appropriate statistical methods, and possessing adequate computational skills. By addressing these challenges and following best practices, researchers can maximize the power of data analysis and derive meaningful insights from their dissertations and capstones.
Recent Comments